Feb, 20-2020 / by - Admin
Data Enrichment Best Practices
Best Practices for Data Enrichment
To maximize the value of data enrichment while maintaining accuracy, security, and compliance, organizations should follow these best practices:
1. Define Clear Objectives
- Why?: Understand the purpose of enrichment. Are you aiming to improve marketing campaigns, enhance customer insights, or boost operational efficiency?
- How?: Set measurable goals, such as increased personalization, better segmentation, or higher data accuracy.
2. Assess Data Quality First
- Why?: Enrichment starts with a solid foundation. Low-quality or incomplete data leads to unreliable results.
- How?: Perform regular data audits to identify duplicates, inconsistencies, and outdated information before enriching.
3. Choose Relevant Data Sources
- Why?: The value of enrichment depends on the quality and relevance of the additional data.
- How?: Use trusted internal and external sources that align with your objectives. For example:
- Internal: CRM systems, historical sales data.
- External: Third-party APIs, demographic databases, or public records.
4. Ensure Data Integration Compatibility
- Why?: Proper integration avoids data silos and inconsistencies.
- How?: Use tools and platforms that support seamless integration across your systems, such as data warehouses or ETL (Extract, Transform, Load) processes.
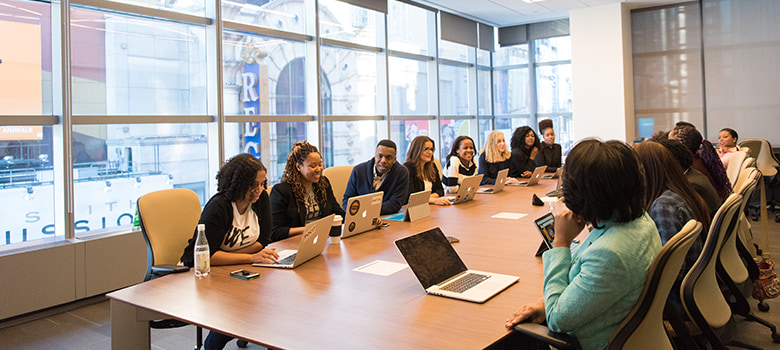
5. Prioritize Data Privacy and Compliance
- Why?: Regulatory frameworks like GDPR, CCPA, and HIPAA impose strict requirements on data usage.
- How?:
- Obtain consent where required.
- Anonymize sensitive data when possible.
- Regularly review compliance policies.
6. Focus on Real-Time or Timely Enrichment
- Why?: Outdated data reduces the effectiveness of decisions.
- How?: Implement real-time enrichment for dynamic use cases (e.g., fraud detection) or schedule periodic updates for static data.
7. Standardize and Normalize Data
- Why?: Enriched data must be usable across all systems.
- How?:
- Apply consistent formatting (e.g., dates, addresses).
- Use standardized taxonomies and classifications.
8. Leverage Automation and AI
- Why?: Manual enrichment is time-consuming and prone to errors.
- How?: Use AI-driven tools and data enrichment platforms to automate processes like:
- Data matching.
- Predictive enrichment (e.g., inferring likely customer interests).
9. Monitor and Maintain Enriched Data
- Why?: Data changes over time, leading to inaccuracies.
- How?:
- Set up regular checks for relevance and accuracy.
- Use automated alerts to identify stale or erroneous data.
10. Balance Depth with Relevance
- Why?: Adding unnecessary data can clutter systems and increase costs.
- How?: Enrich only the data fields that directly align with your goals. For instance, focus on purchase history and preferences for personalization rather than irrelevant attributes.
11. Measure Success and Optimize
- Why?: Continuous improvement ensures enrichment delivers value.
- How?:
- Track KPIs like data accuracy, customer engagement rates, and operational efficiency.
- Solicit feedback from stakeholders to refine enrichment strategies.
By adhering to these best practices, organizations can enhance their data enrichment efforts, ensuring enriched datasets drive actionable insights and meaningful business outcomes.